Khunjerab: Resistive Memory for Machine Learning Applications
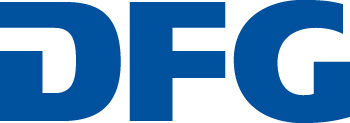
Bridging Applications and Future Emerging Memory for Different Performance-Power-Reliability Trade-Offs
Summary: Resistive RAM (RRAM) is a promising option in the light of diminishing returns from CMOS technology scaling, however, RRAM devices are inherently unstable and require amortization effort to compensate for process variations, wear-off, and stochastic effects during read and write. Khunjerab gears to exploit resistive memory for ML applications, in particular with tradeoffs regarding performance, power consumption, and reliability. In this project we are most interested in codesigning applications and RRAM while not gearing towards upmost reliability, instead we gear to identitfy the most optimal combination within the tuple of performance, power, and reliability (PPR). For instance, some applications might tolerate certain inaccuracies during read and write, while others require that memory operations are certain. It is a joint project with colleagues from Xi’an University of Technology (XAUT), China, the Instiute for Micro-Electronics (IMECAS) at the Chinese Academy of Sciences, the ECML group and the Compute Systems Group (CSG) at Heidelberg University. While XAUT and IMECAS will contribute their experience and expertise in designing innovative RRAM and related devices, CSG will contribute expertise in computer architecture, machine learning, compilers and related compute stack components, and hardware design. Khunjerab has receided funding from the Deutsche Forschungsgemeinschaft (DFG, German national funding, Projektnummer 449797478) and National Natural Science Foundation of China (NSFC), covering a period of three years. The project has started in May 2021 but has been extended recently.
Current people
- Holger Fröning (co-PI)
- Jiawei Zhang (XAUT, co-PI)
- Kai Xi (IMECAS, co-PI)
- Vincent Heuveline (ECML, co-PI)
- Kevin Stehle (PhD student)
- Christian Heusel (bachelor student)
Past people
- Yannick Emonds (PhD student)
Contact
Dissemination
2025
- Implications of Noise in Resistive Memory on Deep Neural Networks for Image ClassificationMachine Learning and Principles and Practice of Knowledge Discovery in Databases, 135–149, Springer Nature Switzerland, 2025
@inproceedings{10.1007/978-3-031-74643-7_11, author = {Emonds, Yannick and Xi, Kai and Fr{\"o}ning, Holger}, editor = {Meo, Rosa and Silvestri, Fabrizio}, title = {Implications of Noise in Resistive Memory on Deep Neural Networks for Image Classification}, booktitle = {Machine Learning and Principles and Practice of Knowledge Discovery in Databases}, year = {2025}, publisher = {Springer Nature Switzerland}, pages = {135--149}, isbn = {978-3-031-74643-7}, url = {https://doi.org/10.1007/978-3-031-74643-7_11}, }
2024
- Random telegraph noise characteristic of nonvolatile resistive random access memories based on optical interference principleJapanese Journal of Applied Physics, 63(3), 031003, IOP Publishing, 2024
@article{Qin_2024, doi = {10.35848/1347-4065/ad26d1}, url = {https://dx.doi.org/10.35848/1347-4065/ad26d1}, year = {2024}, month = mar, publisher = {IOP Publishing}, volume = {63}, number = {3}, pages = {031003}, author = {Qin, Sichen and Zhang, Guiquan and Zhang, Jia-Wei and Zhao, Yu and Song, Chen and Emonds, Yannick and Fröning, Holger}, title = {Random telegraph noise characteristic of nonvolatile resistive random access memories based on optical interference principle}, journal = {Japanese Journal of Applied Physics}, }